“It is a truly shameful vignette of superhuman arrogance and toffishness and twittishness, I suppose. But you know, it was great fun at the time.” — Boris Johnson
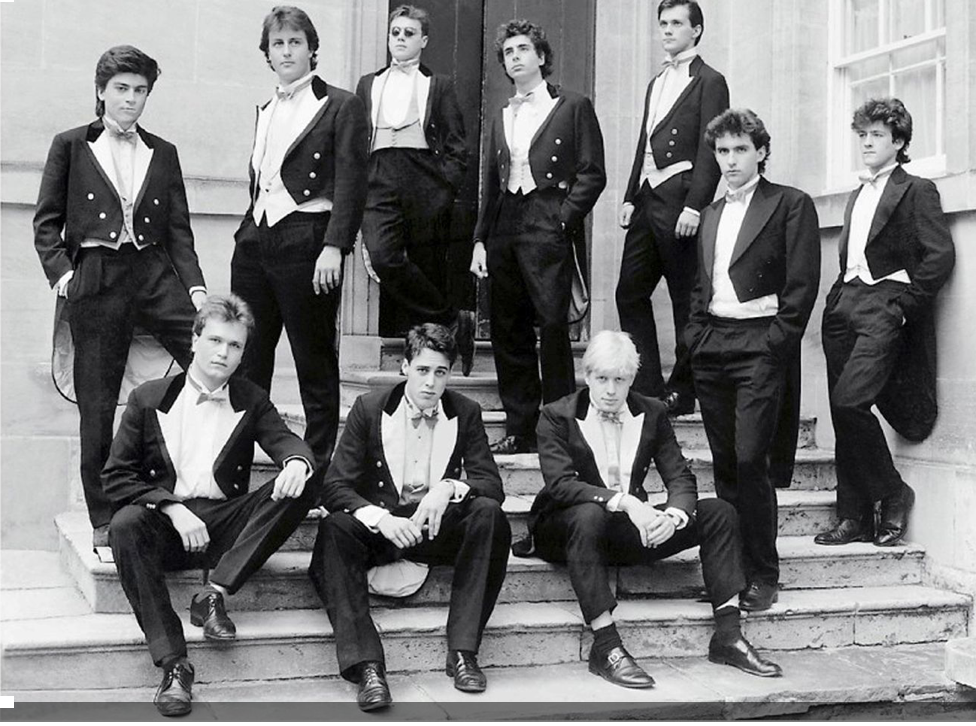
Coffee | Coding | Computers | Church | What does it all mean?
“It is a truly shameful vignette of superhuman arrogance and toffishness and twittishness, I suppose. But you know, it was great fun at the time.” — Boris Johnson
New Scientist once published a ½ page letter in which a working scientist ranted that philosophy was all meaningless and that the Only Worthwhile, And Obviously True, philosophy is Logical Positivism.
But logical positivism is distinguished amongst all philosophies as the one which disproves itself in a 2-line proof.
Logical Positivism Premise #1 : All meaningful statements are either analytic (that is to say, statements of mathematics or logic or some other tautology) or else statements of empirical fact, and any sentence that is not in one of these two categories is strictly and literally meaningless.
2. If premise #1 —which is not a tautology, nor a statement of mathematics or logic, nor a statement of empirical fact—is true, then by premise #1, premise #1 is itself strictly and literally meaningless, so cannot be true.
“I'm sure this is irrelevant chatter but a few days ago I turned on my Nintendo Switch for the first time in months but the battery had drained, so I went to charge it but noticed after 20 minutes it still wouldn't turn on, I tried using another plug and putting it in the dock, it still wasn't working, I knew from early YouTube videos that some people had this problem and sold their console on eBay as "faulty, not accepting charge" when the console was first on the market, not wishing to sell my console I proceeded to calmly take it appart in an attempt to disconnect the battery and plug it in again in order to wake it up, however I'd forgotten how to remove the battery and wasn't sure the tools I had were best for the job, looking at the connector though made me realise if use 2 electrical screwdrivers, I could short the pins, simulating a temporary disconnect, I allowed the battery to spark for a split second and reassembled the unit. Hey presto, the Nintendo Switch is back in full working order, end of story. Good day to you all.”
James Barnes
The hard problem of consciousness, and variations on it, revolves around the difficulty of explaining mental phenomena—I see and smell a rose; I think about my work; I feel pleased by good news—in materialist or physicalist terms.
The presumptive barrier that prevents neurophysiological research answering this question, is that objective observations which can be made by a researcher — such as, electrical current moves through these neurons; a biochemical cascade releases such and such a hormone — appear to be about completely different things than the subjective experiences of a conscious experiencer.
This objective/subjective gap has also been called a first person / third person gap: how can third person sentences such as “that neuron spiked” possibly relate to a first person sentence such as, “I see red.”
Expressed this way, the problem can be studied with formal languages. This appears to make it provably insoluble. It's a hard problem of grammar: there is no sound deduction from a set of 3rd person sentences to a 1st person sentence in any formal logic.
(This claim may not be obvious. It's like claiming that some form of the Church-Turing thesis applies not only to mathematics and logic, but to rational discourse more widely, including empirical research. I'm suggesting that any argument which is genuinely rational, can be expressed in a formal language. If some part of the argument can't be formalised then I think we will discover, on inspection, that it's because the argument isn't rational. Either it will be a non-sequitor, or it will be an appeal to emotion, or an ad hominem attack, or somesuch).
(This is essentially an assertion that some form of the Craig interpolation theorem can be proven for any formal grammar suitable for rational discourse. The Craig interpolation theorem says (more-or-less), that given a set of sentences only about apples, you cannot validly deduce from them a sentence about oranges. I suggest that any formal language that does not satisfy this constraint is not a language we can use for rational discourse. It may still be fine for poetry; but not for being rational).
I think any attempt to define 1st & 2nd person words – I, you, me, we – in 3rd person terms fails. Any definition using only 3rd person terms can only succeed in defining 3rd person 'things'.
The thought is somewhat similar to Chalmer's “Structure and functions” argument: anything you can define with structure and function will itself be structure and function. I think the grammatical argument is stronger, surprisingly (one doesn't expect arguing about grammar to prove anything!), because 1st and 2nd person speech and relationships is, and always has been, a core part of the reality of human experience.
Intuitively, throughout the modern era, people have always felt that a reductionist materialist account of humanity surely misses something. The grammar of every human language (at least, every one that I know of) embodies that fact.